Machine learning is reshaping industries and enhancing everyday life through its diverse applications. From improving healthcare outcomes and financial services to revolutionizing retail and transportation, the impact of ML is profound and far-reaching. As technology continues to advance, we can expect even more innovative applications and solutions, driving progress and transforming the way we live and work.
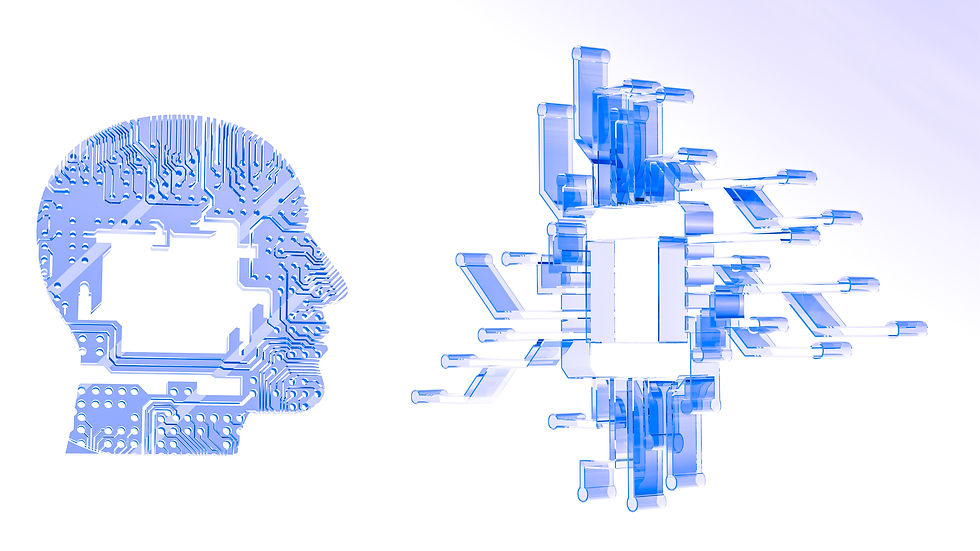
What is Machine Learning? Its Significance & Importance.
Machine learning (ML) is a subset of artificial intelligence (AI) that focuses on the development of algorithms and statistical models that enable computers to learn from and make predictions or decisions based on data. Unlike traditional programming, where explicit instructions are given to perform a task, machine learning allows systems to improve their performance autonomously by recognizing patterns and learning from experiences. This capability has revolutionized numerous industries by providing sophisticated data analysis, predictive modeling, and automation. The significance of machine learning lies in its ability to handle large volumes of data, uncover insights that are not immediately apparent, and adapt to new information without human intervention. Its importance extends to enhancing decision-making processes, personalizing user experiences, optimizing operations, and driving innovation across various domains. Fundamentals of Machine Learning
Data Collection: Gathering and preparing relevant data from various sources to train and validate models.
Data Preprocessing: Cleaning and transforming raw data to ensure it is suitable for analysis, including handling missing values and normalizing data.
Feature Engineering: Selecting and creating features (input variables) that are most relevant for making accurate predictions or classifications.
Model Selection: Choosing appropriate algorithms and techniques based on the problem type (e.g., classification, regression, clustering).
Training: Using labeled data to teach the model how to make predictions or decisions by adjusting parameters to minimize error.
Validation: Assessing model performance on a separate dataset to ensure it generalizes well to new, unseen data and avoids overfitting.
Evaluation: Measuring the model’s accuracy and effectiveness using metrics such as precision, recall, F1 score, and ROC-AUC.
Hyperparameter Tuning: Optimizing model parameters to enhance performance and efficiency.
Deployment: Integrating the trained model into real-world applications and systems for practical use.
Monitoring and Maintenance: Continuously evaluating the model’s performance and updating it as needed to adapt to new data or changes in the environment.
Applications of Machine Learning
Machine learning (ML) has rapidly evolved from a niche area of artificial intelligence into a transformative technology impacting numerous sectors. Its ability to analyze vast amounts of data, learn from patterns, and make predictions or decisions has led to groundbreaking applications across various fields. Here’s a look at some of the most impactful applications of machine learning today.
1. Machine Learning in Healthcare
In healthcare, machine learning is revolutionizing diagnostics and patient care. Algorithms can analyze medical images, such as MRIs and X-rays, to detect anomalies with high accuracy. For example, ML models are used to identify early signs of diseases like cancer or diabetic retinopathy, often outperforming human experts. Additionally, ML aids in personalized medicine by analyzing genetic data to tailor treatments to individual patients. Predictive analytics also helps in forecasting patient admissions and optimizing hospital resources.
2. Machine Learning in Finance
The finance industry utilizes machine learning for various purposes, from fraud detection to algorithmic trading. ML models analyze transaction patterns to identify potentially fraudulent activities, significantly reducing financial crime. In trading, algorithms execute high-frequency trades based on real-time data analysis, maximizing returns and minimizing risks. Moreover, ML-powered tools assist in credit scoring and risk assessment, improving the accuracy of financial predictions and decision-making.
3. Machine Learning in Retail and E-commerce
In retail and e-commerce, machine learning enhances customer experiences and optimizes operations. Recommendation systems, like those used by Amazon and Netflix, analyze user behavior and preferences to suggest products or content tailored to individual tastes. ML algorithms also forecast demand, manage inventory, and optimize pricing strategies. Additionally, chatbots powered by natural language processing (NLP) provide personalized customer service, improving engagement and satisfaction.
4. Machine Learning Transportation
Machine learning is at the heart of advancements in autonomous vehicles and transportation systems. Self-driving cars use ML algorithms to process data from sensors and cameras, making real-time decisions to navigate roads safely. In public transportation, ML models optimize routes and schedules, enhancing efficiency and reducing operational costs. Additionally, predictive maintenance powered by ML helps in anticipating vehicle breakdowns, minimizing downtime, and extending the lifespan of assets.
5. Machine Learning in Agriculture
Agriculture is benefiting from machine learning through precision farming techniques. ML algorithms analyze data from satellite imagery, soil sensors, and weather forecasts to optimize crop management practices. This includes predicting crop yields, detecting plant diseases early, and improving irrigation practices. By leveraging ML, farmers can make data-driven decisions that enhance productivity, reduce waste, and promote sustainable farming.
6. Machine Learning in Education
In education, machine learning is transforming teaching and learning experiences. Adaptive learning platforms use ML to tailor educational content to individual students’ needs, providing personalized instruction and feedback. Additionally, ML-powered tools assist in automating administrative tasks, such as grading and scheduling. Predictive analytics can identify students at risk of falling behind, enabling timely interventions to support their academic success.
7. Machine Learning in Entertainment
Machine learning has a significant impact on the entertainment industry, from content creation to audience engagement. Algorithms analyze viewing habits and preferences to recommend movies, music, or games that align with user interests. ML is also used in content generation, such as creating realistic graphics and animations in video games or generating music and art. Additionally, sentiment analysis helps in understanding audience reactions and improving marketing strategies.
Conclusion
In conclusion, machine learning represents a transformative leap in technology, offering unprecedented capabilities to analyze, interpret, and act on vast amounts of data. By enabling systems to learn from experiences and adapt over time, machine learning not only enhances decision-making but also drives efficiency, personalization, and innovation across a multitude of sectors. Its impact is evident in diverse fields such as healthcare, finance, retail, transportation, agriculture, education, and entertainment, where it continually reshapes how we approach problems and opportunities. The fundamentals of machine learning—including data collection, preprocessing, feature engineering, model selection, training, validation, evaluation, hyperparameter tuning, deployment, and ongoing maintenance—provide a robust framework for developing and implementing effective ML solutions. As technology advances, the potential for machine learning to further revolutionize industries and improve daily life remains vast. Embracing and understanding these fundamentals is crucial for harnessing the full power of machine learning, ensuring that its applications are both innovative and beneficial.
.
Comments