Unveiling the Power of Big Data Analytics
- Samul Black
- Jan 10, 2024
- 6 min read
Let's delve into the realm of big data analytics, exploring its significance, techniques, applications, and future trends.
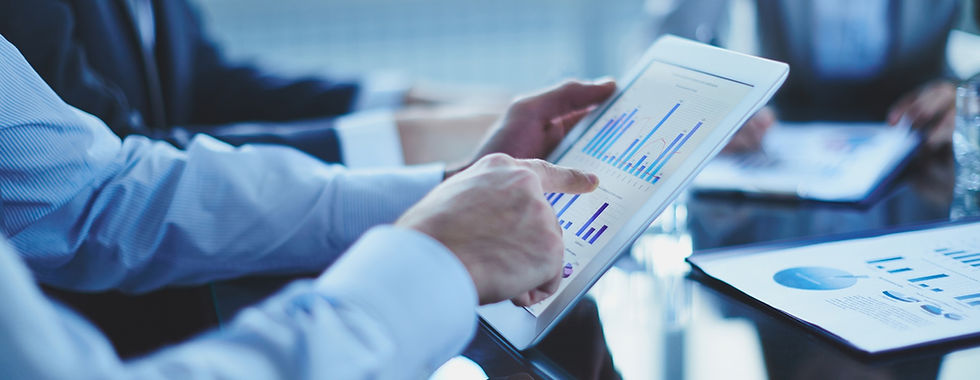
What is Big Data?
In today's digital age, the vast amounts of data generated and collected present both opportunities and challenges for businesses and industries worldwide. This avalanche of data, often referred to as "big data," has transformed how organizations operate, make decisions, and innovate. Big data analytics represents the transformative force driving modern businesses and industries, harnessing the immense volume, velocity, variety, and veracity of data to extract valuable insights. It encompasses a spectrum of techniques and technologies aimed at deciphering complex datasets, revealing patterns, trends, and correlations that traditional analytics might overlook. This analytical approach involves not just processing massive amounts of data but also leveraging sophisticated algorithms, machine learning models, and visualization tools to make sense of this information. From descriptive analytics, providing a retrospective view of past data, to predictive analytics, forecasting future trends, and prescriptive analytics, guiding actionable decisions, big data analytics empowers organizations to optimize operations, innovate products, enhance customer experiences, and drive strategic decision-making in an ever-evolving digital landscape. Its applications span across industries, revolutionizing healthcare, finance, retail, manufacturing, and more, ushering in an era where data isn't just an asset but the key to unlocking insights for growth and competitiveness.Big data is characterized by four key aspects: volume, velocity, variety, and veracity. Volume refers to the sheer amount of data, often ranging from terabytes to petabytes. Velocity signifies the speed at which data is generated and needs to be processed. Variety encompasses the diverse types of data, from structured to unstructured sources like text, images, and sensor data. Veracity emphasizes the need for accurate and reliable data for effective analysis.
Big Data Analytics Techniques
Big data analytics comprises various techniques, including descriptive, predictive, and prescriptive analytics. Big data analytics techniques encompass various methodologies and tools aimed at extracting meaningful insights from large and complex datasets. These techniques and methodologies, often used in combination, empower organizations to derive actionable insights, optimize decision-making processes, and uncover valuable opportunities from the vast volumes of data available in today's digital landscape. Some prominent techniques include:
Descriptive Analytics
This technique involves summarizing historical data to gain an understanding of past trends and patterns. It utilizes tools like aggregation, data mining, and visualization to provide a comprehensive view of what has happened within the data.
Predictive Analytics
Predictive analytics uses statistical algorithms and machine learning models to forecast future outcomes based on historical data patterns. It aids in identifying trends, making predictions, and assessing potential risks or opportunities.
Prescriptive Analytics
Going beyond predicting future events, prescriptive analytics suggests potential actions and strategies to optimize outcomes. It employs advanced algorithms, optimization techniques, and simulation models to recommend the best course of action to achieve desired goals.
Machine Learning Algorithms
Within big data analytics, machine learning algorithms play a crucial role. These algorithms, including decision trees, neural networks, clustering, and regression, enable systems to learn from data and make predictions or decisions without explicit programming.
Natural Language Processing (NLP)
NLP is a technique that enables computers to interpret and analyze human language. It's used in sentiment analysis, text mining, and language translation, allowing for insights from unstructured textual data.
Data Mining
Data mining involves discovering patterns and relationships within large datasets. It utilizes techniques like clustering, association rule mining, and anomaly detection to uncover hidden insights from structured data.
Time Series Analysis
This technique focuses on analyzing sequences of data points collected over time. It includes methods to understand trends, seasonal variations, and cyclic patterns within time-stamped data.
Graph Analytics
Graph analytics deals with analyzing and visualizing relationships and connections within datasets represented as graphs or networks. It helps in understanding complex relationships and identifying influential nodes within networks.
Tools and Technologies
Several tools and technologies drive big data analytics. Apache Hadoop, Apache Spark, and Apache Flink are popular frameworks for distributed storage and processing of large datasets. Data visualization tools like Tableau and Power BI assist in presenting complex insights in a comprehensible manner. Additionally, machine learning algorithms and AI contribute significantly to analyzing and deriving insights from vast datasets.
Different Sources of Data for Big Data Analytics
Big data analytics relies on various sources to gather and analyze vast amounts of data. Utilizing diverse sources of data and combining them through data integration and aggregation techniques forms the foundation for comprehensive big data analytics, enabling organizations to derive valuable insights and make data-driven decisions.Some key sources of data for big data analytics include:
Transactional Data: Information generated from daily business transactions, such as sales records, customer orders, financial transactions, and inventory logs.
Social Media Data: Data extracted from social networking platforms, including user interactions, comments, likes, shares, and posts. This data provides insights into customer sentiments, behavior, and trends.
Sensor and IoT Data: Information collected from sensors, connected devices, and the Internet of Things (IoT). This includes data from smart devices, wearables, industrial sensors, and environmental sensors, offering real-time insights into various applications like healthcare, manufacturing, and smart cities.
Web and Clickstream Data: Data generated from web interactions, website visits, clicks, navigation paths, and user behavior on websites or applications. It's valuable for understanding user preferences, optimizing user experiences, and analyzing web traffic patterns.
Machine and Log Data: Data logs produced by machines, servers, networks, and applications. It includes system logs, error logs, event logs, and performance metrics, aiding in troubleshooting, system monitoring, and optimization.
Geospatial Data: Geographic data obtained from GPS, satellites, maps, and geographic information systems (GIS). Geospatial data helps in location-based analytics, route optimization, urban planning, and environmental monitoring.
Textual and Unstructured Data: Unstructured data from emails, documents, surveys, and text sources. Natural Language Processing (NLP) and text mining techniques analyze this data for sentiment analysis, categorization, and extracting valuable insights.
Financial and Market Data: Data related to financial markets, stock exchanges, economic indicators, and market trends. Analyzing this data aids in financial forecasting, risk assessment, and investment strategies.
Biometric and Health Data: Health-related data from wearable devices, medical records, genomic data, and biometric sensors. It's utilized for personalized medicine, healthcare analytics, and disease prediction.
Audio and Video Data: Multimedia data including audio recordings, video streams, and images. Techniques like computer vision and speech recognition analyze this data for pattern recognition, object detection, and content analysis.
Applications of Big Data Analytics
The applications of big data analytics span various domains. In business intelligence, it aids in understanding customer behavior, optimizing supply chains, and making data-driven decisions. In healthcare, it revolutionizes patient care, disease prediction, and drug discovery. Financial institutions leverage big data analytics for risk assessment, fraud detection, and portfolio management. These applications showcase the broad spectrum of big data analytics, highlighting its transformative impact across industries, driving innovation, and enabling data-driven decision-making for a more efficient and competitive landscape. Big data analytics finds applications across diverse industries, revolutionizing how organizations operate, make decisions, and innovate. Some key applications include:
Business Intelligence and Analytics: Big data analytics empowers businesses to gain insights into customer behavior, market trends, and operational efficiency. It aids in optimizing processes, enhancing product offerings, and identifying new business opportunities.
Healthcare Analytics: In healthcare, big data analytics improves patient outcomes by analyzing electronic health records (EHRs), medical imaging data, and patient-generated data. It aids in personalized medicine, disease prediction, and optimizing healthcare delivery.
Financial Services: In the financial sector, big data analytics is crucial for risk assessment, fraud detection, algorithmic trading, and customer relationship management. It helps in making informed investment decisions and complying with regulatory requirements.
Retail and E-commerce: Big data analytics drives personalized marketing strategies, customer segmentation, and recommendation systems in retail and e-commerce. It optimizes pricing strategies, inventory management, and enhances the overall shopping experience.
Manufacturing and Supply Chain: In manufacturing, analytics optimizes production processes, predictive maintenance, and quality control by analyzing sensor data from machines. It also aids in supply chain optimization, demand forecasting, and inventory management.
Telecommunications and Networking: Big data analytics assists telecom companies in analyzing network traffic, optimizing service quality, and predicting network failures. It helps in providing better services and improving network infrastructure.
Smart Cities and Urban Planning: Cities utilize big data analytics for urban planning, traffic management, waste management, and energy consumption optimization. It enhances public services, improves infrastructure, and fosters sustainability.
Energy and Utilities: In the energy sector, big data analytics optimizes energy generation, distribution, and consumption. It enables predictive maintenance of equipment, monitors energy usage patterns, and supports renewable energy integration.
Education and Research: Big data analytics aids in educational institutions by personalizing learning experiences, improving student outcomes, and optimizing administrative operations. In research, it facilitates data-driven discoveries and insights.
Cybersecurity: Big data analytics strengthens cybersecurity by analyzing patterns in network traffic, detecting anomalies, and identifying potential threats or security breaches.
Ethical Considerations
Amidst the vast potential of big data analytics, ethical considerations and data privacy are paramount. Responsible handling of sensitive information and ethical use of data are crucial for maintaining trust and compliance with regulations.
In conclusion, big data analytics has become a cornerstone of modern businesses and industries, empowering decision-makers with insights and strategies to navigate an increasingly data-driven world. Embracing this technology responsibly and innovatively will continue to drive advancements and shape the future of industries and societies. Looking ahead, edge computing is poised to play a significant role by processing data closer to its source, reducing latency and bandwidth usage. Advancements in AI and machine learning will further enhance the capabilities of big data analytics, paving the way for more accurate predictions and intelligent automation.
Comments