Impact of Business Analytics: What is Business Analytics? its importance, applications, types and components.
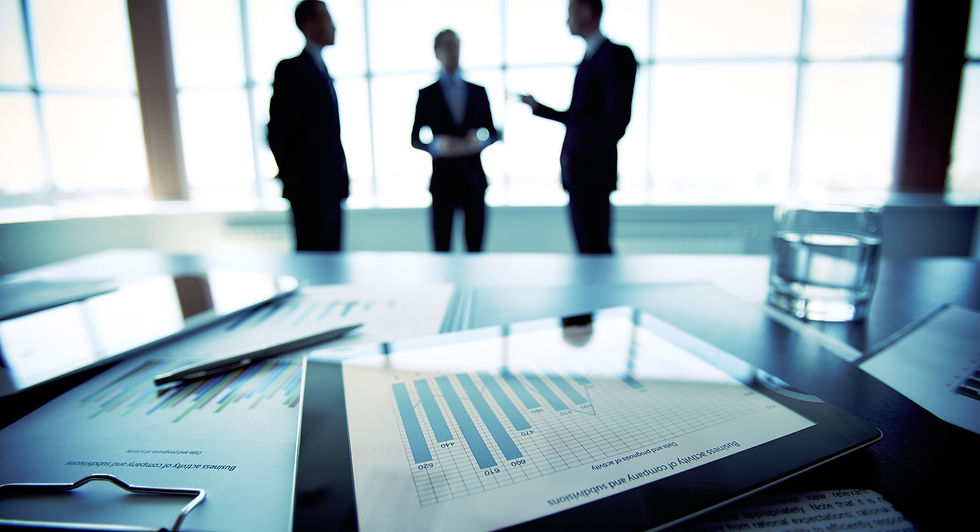
What is business Analytics?
Business analytics refers to the systematic exploration, interpretation, and utilization of data to derive actionable insights that drive informed decision-making within an organization. It involves the application of statistical analysis, data mining, predictive modeling, and other analytical techniques to extract meaningful patterns, correlations, and trends from diverse datasets. The primary aim of business analytics is to transform raw data into valuable insights, facilitating a deeper understanding of business operations, customer behavior, market trends, and other crucial aspects. By leveraging data-driven insights, businesses can optimize strategies, improve operational efficiency, identify opportunities, mitigate risks, and enhance overall performance across various departments and functions. Ultimately, business analytics empowers organizations to make evidence-based decisions, foster innovation, and gain a competitive edge in a rapidly evolving business landscape.
Importance of Data in Business Analytics
In today's competitive business landscape, the significance of data-driven insights cannot be overstated. Data serves as the lifeblood of modern businesses, offering a wealth of information that organizations can leverage to gain a competitive edge. Here's why data-driven insights are crucial:
Informed Decision-Making: Data provides the foundation for informed decision-making. It empowers business leaders to base their strategies, plans, and actions on concrete evidence rather than intuition or guesswork.
Understanding Customers: Data analytics allows businesses to understand their customers on a deeper level. It provides insights into customer preferences, behaviors, and sentiments, enabling the customization of products, services, and marketing strategies to better meet their needs.
Operational Efficiency: Analyzing operational data helps optimize processes, streamline workflows, and identify bottlenecks or inefficiencies. This leads to cost savings, improved productivity, and enhanced overall efficiency.
Predictive Insights: Utilizing historical and real-time data enables predictive analytics, which forecasts future trends, market changes, and potential risks. This proactive approach allows businesses to prepare for challenges and capitalize on opportunities before they arise.
Innovation and Growth: Data-driven insights often uncover new opportunities and areas for innovation. By analyzing market trends, consumer behavior, and emerging technologies, businesses can innovate and stay ahead in rapidly evolving industries.
Competitive Advantage: Organizations that effectively harness data have a distinct advantage over competitors. They can swiftly adapt to market changes, personalize customer experiences, and make strategic decisions based on a comprehensive understanding of their business environment.
Customer-Centric Approach: Data-driven insights facilitate a customer-centric approach, allowing businesses to anticipate customer needs, offer personalized experiences, and build long-lasting relationships, fostering customer loyalty.
Risk Mitigation: Analyzing data helps identify and mitigate risks, whether they're related to cybersecurity, market fluctuations, operational challenges, or compliance issues.
In essence, data-driven insights drive innovation, improve operational efficiency, enhance customer experiences, and enable businesses to stay agile and responsive in an increasingly dynamic and competitive marketplace. They serve as a catalyst for growth and sustainability, empowering organizations to navigate challenges and capitalize on opportunities in a rapidly evolving business landscape.
Types of Business Analytics
Each type of business analytics plays a distinct role in analyzing data and deriving insights at different levels of understanding. Here's an overview of the key types:
Descriptive Analytics:
Descriptive analytics focuses on summarizing historical data to provide an understanding of past events or trends. It deals with what has happened, offering insights into the current state of affairs. This type of analytics utilizes various statistical techniques, data aggregation, and visualization tools to present data in a meaningful and easily interpretable format. It includes reports, dashboards, KPIs, and data visualizations to represent trends, patterns, and key metrics.
Diagnostic Analytics:
Diagnostic analytics delves deeper into the data to understand why specific events occurred. It investigates the causes behind historical trends or occurrences. This involves root cause analysis, data mining techniques, correlation analysis, and drill-down capabilities to identify patterns and relationships within the data. By analyzing the "why" behind past outcomes, it helps in understanding the factors influencing those outcomes.
Predictive Analytics:
Predictive analytics forecasts future outcomes based on historical data patterns. It identifies trends, patterns, and relationships within the data to make predictions about future events. Machine learning algorithms, regression analysis, time series forecasting, and data modeling are employed to predict future trends, behaviors, or outcomes. This type of analytics aids in anticipating future scenarios and making proactive decisions.
Prescriptive Analytics:
Prescriptive analytics goes beyond predicting future events by suggesting the best course of action to achieve desired outcomes. It provides recommendations on what actions to take based on predictive models. Optimization algorithms, simulation models, and decision-making frameworks are used to generate actionable insights. It helps in determining the best strategies, interventions, or decisions to optimize outcomes.
These types of analytics are often viewed as a continuum, with descriptive analytics laying the foundation by summarizing data, diagnostic analytics investigating why things happened, predictive analytics forecasting what might happen, and prescriptive analytics guiding what actions should be taken. By employing these types of analytics in tandem, organizations can gain a comprehensive understanding of their data, derive valuable insights, and make more informed and strategic decisions to drive business success.
Components of Business Analytics
The components of business analytics encompass various stages and methodologies that contribute to deriving actionable insights from data. These components collectively form the backbone of business analytics, facilitating the process of transforming raw data into valuable insights that drive informed decision-making and strategic initiatives within organizations. Each stage contributes to uncovering patterns, making predictions, and guiding actionable strategies based on data-driven evidence.
Data Collection: Data collection forms the foundation of business analytics, involving the gathering of diverse datasets from various sources. This component encompasses acquiring data from internal systems like databases and external sources such as social media platforms or market research databases. Effective data collection strategies ensure the availability of comprehensive and relevant datasets necessary for analysis, decision-making, and deriving insights.
Data Preprocessing and Cleaning: Before analysis, data often requires preprocessing and cleaning to ensure accuracy and consistency. This stage involves handling missing values, removing duplicates, standardizing formats, and addressing outliers or errors. By employing techniques like data wrangling and normalization, businesses ensure that the data is prepared appropriately for subsequent analysis.
Data Mining: Data mining plays a crucial role in uncovering hidden patterns, correlations, or relationships within large datasets. This component involves using various techniques like clustering, classification, association rule mining, and anomaly detection to extract valuable information and insights from raw data. Data mining aids in discovering meaningful patterns that contribute to informed decision-making.
Statistical Analysis: Statistical analysis employs statistical techniques to interpret and validate findings, quantify relationships, and make data-driven inferences. Techniques like hypothesis testing, regression analysis, and correlation analysis aid in understanding patterns and relationships within the data, providing a deeper understanding of trends and behaviors.
Predictive Modeling: Predictive modeling utilizes historical and current data to forecast future trends or outcomes. This component involves building models using machine learning algorithms, regression models, decision trees, and neural networks. Predictive modeling assists in making informed predictions and anticipating future events based on patterns identified within the data.
Visualization Techniques: Visualization techniques are essential for presenting complex data in an easily understandable and visually appealing format. This component employs tools like charts, graphs, dashboards, and interactive visualizations to represent data effectively. Visualizations aid stakeholders in interpreting trends, patterns, and relationships within the data, facilitating quicker and more informed decision-making.
These components collectively form the core of business analytics, guiding the process of transforming raw data into actionable insights that drive strategic decisions and enhance business performance. Each component plays a vital role in different stages of the analytics process, contributing to the comprehensive analysis and interpretation of data for informed decision-making within organizations.
Applications of Business Analytics:
Business analytics finds applications across diverse industries and functional areas, empowering organizations to leverage data-driven insights for strategic decision-making. Here are some prominent applications:
Marketing and Customer Analytics:
Segmentation and Targeting: Business analytics assists in segmenting customers based on behavior, demographics, and preferences. It aids in targeted marketing strategies and personalized campaigns.
Customer Retention: Analytics helps identify at-risk customers, predict churn rates, and implement retention strategies by understanding customer needs and behaviors.
Operations and Supply Chain Management:
Process Optimization: Analytics optimizes operational processes, reduces inefficiencies, and improves supply chain management by analyzing production data, inventory levels, and logistics.
Demand Forecasting: Predictive analytics forecasts demand, enabling businesses to manage inventory levels efficiently and meet customer needs.
Financial Analysis and Risk Management:
Risk Assessment: Business analytics assists in risk assessment and management by analyzing financial data, market trends, and identifying potential risks in investments or operations.
Fraud Detection: Analytics tools and models help detect anomalies and patterns indicating fraudulent activities, safeguarding businesses against financial losses.
Human Resources and Workforce Management:
Talent Acquisition: Analytics aids in identifying and attracting top talent by analyzing resumes, job postings, and candidate data.
Performance Management: It helps evaluate employee performance, identify training needs, and optimize workforce planning.
Healthcare Analytics:
Patient Care and Treatment: Analytics improves patient outcomes by analyzing medical records, treatment plans, and patient data to personalize treatments and predict potential health issues.
Operational Efficiency: It optimizes hospital operations by analyzing resource allocation, patient flow, and healthcare costs.
Retail and E-commerce:
Inventory Management: Analytics optimizes inventory levels, predicts demand, and ensures stock availability by analyzing sales data and consumer behavior.
Recommendation Systems: Personalized recommendations based on past purchases and browsing behavior enhance the customer experience.
Telecommunications and Networking:
Network Optimization: Analytics optimizes network performance, predicts network failures, and manages bandwidth by analyzing network data and traffic patterns.
Customer Service Improvement: Analyzing customer feedback and service data helps improve service quality and customer satisfaction.
Smart Cities and Urban Planning:
Urban Infrastructure: Analytics aids in city planning, traffic management, energy consumption optimization, and waste management by analyzing data from IoT devices and sensors.
Business analytics serves as a strategic tool across industries, enabling organizations to optimize processes, enhance decision-making, and gain a competitive advantage by leveraging data-driven insights. Its applications continue to evolve, driving innovation and efficiency across various business functions and sectors.
Impact of Business Analytics on Modern Business Practices
Business analytics has fundamentally transformed modern business practices by revolutionizing how organizations collect, analyze, and leverage data to drive strategic decision-making. Its impact can be summarized in several key aspects:
Informed Decision-Making: Business analytics empowers businesses to make informed decisions backed by data-driven insights rather than relying solely on intuition or past experiences. This shift leads to more accurate and strategic decision-making across all facets of an organization.
Operational Efficiency: By analyzing operational data, organizations optimize processes, identify inefficiencies, and streamline workflows. This results in improved productivity, cost reductions, and overall operational excellence.
Enhanced Customer Experiences: Through customer analytics, businesses gain deeper insights into consumer behavior, preferences, and needs. This enables personalized marketing strategies, tailored products/services, and improved customer satisfaction and retention.
Predictive Capabilities: Predictive analytics forecasts future trends, market changes, and potential risks by analyzing historical data patterns. This proactive approach allows businesses to anticipate challenges and capitalize on opportunities before they arise.
Innovation and Agility: Business analytics fosters a culture of innovation by uncovering new opportunities and areas for improvement. It enables organizations to adapt quickly to market changes and technological advancements, fostering agility in decision-making and strategy implementation.
Competitive Advantage: Organizations that effectively utilize business analytics gain a significant competitive edge. They can swiftly adapt to market shifts, personalize offerings, and make strategic decisions based on a comprehensive understanding of their business environment.
Continuous Improvement: Analytics facilitates a cycle of continuous improvement by providing real-time feedback and insights. This iterative process allows businesses to refine strategies, products, and services based on evolving market dynamics and customer preferences.
In essence, the transformative impact of business analytics lies in its ability to turn raw data into actionable insights, guiding organizations toward greater efficiency, innovation, and competitiveness in a dynamic and data-driven business landscape. It fundamentally changes the way businesses operate, strategize, and thrive in today's rapidly evolving environment.
コメント