Data Analytics: A brief account of data analytics with its significance and relevance in todays data driven world along with its different types, applications and components.
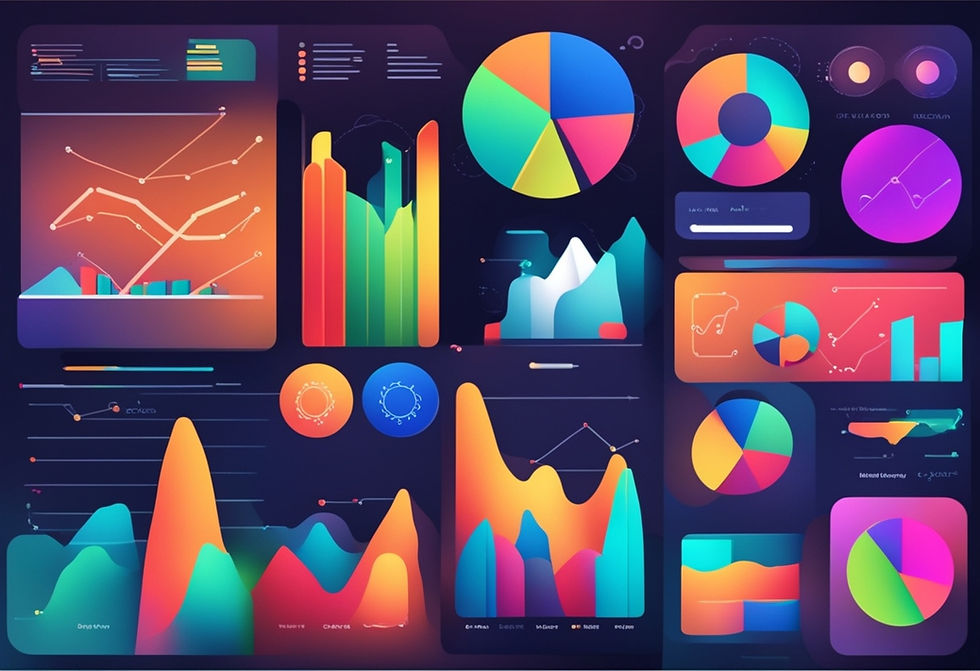
Data analytics plays a pivotal role in driving efficiency, innovation, and progress across industries, influencing how organizations operate, compete, and evolve in our data-centric era.
Brief Explanation of Data Analytics
Data analytics involves the systematic exploration, interpretation, and transformation of raw data into meaningful insights, patterns, and trends. It employs various statistical, mathematical, and computational techniques to analyze large datasets, uncover hidden patterns, and extract valuable information that aids decision-making processes. By utilizing tools, technologies, and methodologies, data analytics allows organizations to derive actionable insights, optimize operations, predict future trends, and drive strategic planning. Ultimately, it empowers businesses and industries to make informed decisions, solve complex problems, and gain a competitive edge in today's data-driven world.
Significance of Data Analytics in Today's Data-Driven World
In today's data-driven world, data analytics holds immense significance as it empowers organizations to harness the vast amounts of data generated daily. Its significance lies in several key aspects:
Informed Decision-Making:
Data analytics provides the means to extract valuable insights from large datasets. These insights enable businesses to make informed, evidence-based decisions, guiding strategies, and actions for optimal outcomes.
Business Optimization:
By analyzing data, organizations can identify inefficiencies, optimize processes, and improve overall operational performance. This leads to enhanced productivity, cost reduction, and streamlined workflows.
Competitive Advantage:
Effective use of data analytics gives businesses a competitive edge. Understanding market trends, customer behaviour, and predictive insights allows organizations to innovate and adapt swiftly to changing landscapes.
Personalization and Customer Experience:
Data analytics aids in understanding customer preferences and behavior, facilitating personalized services and products. This enhances customer satisfaction, loyalty, and engagement.
Innovation and Future Planning:
Insights gleaned from data analytics help in forecasting trends, anticipating market shifts, and fostering innovation. It aids in proactive planning and strategy for future growth.
Healthcare and Research:
In fields like healthcare and scientific research, data analytics drives advancements, improving patient care, discovering new treatments, and enabling breakthroughs through data-driven insights.
Societal Impact:
Data analytics influences various sectors, contributing to better policy making, urban planning, resource allocation, and addressing societal challenges through data-backed solutions.
Relevance of Data Analytics
Data analytics is the systematic process of examining raw data with the goal of extracting meaningful insights, patterns, and trends. It involves various techniques, including statistical analysis, machine learning, and data mining, to derive actionable information from large datasets. Its relevance lies in its ability to convert data into valuable insights that drive informed decision-making, operational efficiency, and strategic planning across industries. By analyzing and interpreting data, organizations gain a deeper understanding of trends, customer behaviour, market dynamics, and operational performance, enabling them to make data-driven decisions, optimize processes, and gain a competitive edge in a rapidly evolving business landscape. Ultimately, data analytics empowers organizations to unlock the potential hidden within their data, turning it into a valuable asset for growth and innovation.
Types of Data Analytics
Each type of data analytics serves distinct purposes and offers unique insights, collectively contributing to informed decision-making, proactive strategies, and operational optimization within organizations. Data analytics encompasses various approaches to analyzing and interpreting data. Here are the main types of data analytics:
1. Descriptive Analytics:
Descriptive analytics focuses on understanding historical data to describe what has happened in the past. It involves summarizing, organizing, and visualizing data to gain insights into trends, patterns, and key performance indicators (KPIs). This type of analytics helps in understanding the current state of affairs.
2. Diagnostic Analytics:
Diagnostic analytics aims to delve deeper into data to understand the reasons behind past events or outcomes. It involves examining data to identify correlations, causations, and relationships, allowing organizations to diagnose the root causes of specific occurrences or trends.
3. Predictive Analytics:
Predictive analytics utilizes statistical algorithms and machine learning techniques to forecast future outcomes based on historical data. By analyzing patterns and trends, predictive analytics helps in making predictions about potential future events or behaviours, enabling proactive decision-making.
4. Prescriptive Analytics:
Prescriptive analytics goes beyond predicting future outcomes by suggesting actions or interventions to optimize results. It utilizes advanced algorithms to recommend the best course of action based on predictive models, helping organizations make informed decisions for optimal results.
5. Real-time Analytics:
Real-time analytics involves analyzing data as it is generated or received, allowing immediate insights and decision-making. This type of analytics is crucial in situations where quick responses or immediate actions are necessary, such as in financial trading or cybersecurity.
Key Components of Data Analytics
Data analytics involves several key components that collectively contribute to the process of deriving insights from data:
1. Data Collection:
This initial phase involves gathering raw data from various sources, including databases, sensors, IoT devices, social media, and more. The data collected can be structured (e.g., databases) or unstructured (e.g., text, images), requiring different methods of handling and processing.
2. Data Preprocessing and Cleaning:
Raw data often contains inconsistencies, errors, or missing values. Data preprocessing involves cleaning, transforming, and organizing the data to ensure its quality and readiness for analysis. This step includes handling missing values, removing duplicates, and standardizing formats.
3. Data Storage and Management:
Data needs to be stored in a manner that facilitates efficient access, retrieval, and analysis. Data storage systems, databases, data warehouses, or cloud-based solutions are utilized to securely manage and store vast amounts of data.
4. Data Analysis Techniques:
Various statistical, mathematical, and computational techniques are employed to analyze data. This includes descriptive statistics, regression, clustering, classification, machine learning algorithms, and more, depending on the objectives of analysis.
5. Visualization and Interpretation:
Data visualization tools and techniques help present complex data in an understandable format. Visualizations such as charts, graphs, dashboards, and interactive displays aid in understanding patterns, trends, and relationships within the data.
6. Interpretation and Decision-making:
The insights derived from data analysis are interpreted to extract meaningful information. These insights play a crucial role in informed decision-making, guiding strategies, actions, and future planning within organizations.
7. Iterative Process:
Data analytics is often an iterative process, wherein analysis, interpretation, and decision-making lead to further data collection, refinement of models, and continuous improvement in analytics strategies.
Collectively, these components form the foundation of data analytics, enabling organizations to extract valuable insights and derive actionable information from their data to drive informed decision-making and achieve business objectives.
Applications of Data Analytics Across Industries
Data analytics finds application across a wide spectrum of industries, revolutionizing operations and decision-making processes. Here are some notable applications:
1. Business and Marketing:
Market Segmentation and Customer Profiling
Personalized Marketing Campaigns
Sales Forecasting and Demand Prediction
2. Healthcare:
Disease Diagnosis and Predictive Analytics
Patient Monitoring and Personalized Medicine
Drug Development and Clinical Trials Optimization
3. Finance and Banking:
Fraud Detection and Risk Management
Credit Scoring and Loan Approvals
Algorithmic Trading and Market Analysis
4. Manufacturing and Supply Chain:
Predictive Maintenance and Equipment Optimization
Inventory Management and Demand Forecasting
Supply Chain Optimization and Logistics
5. Telecommunications:
Network Optimization and Predictive Maintenance
Customer Churn Prediction and Retention Strategies
Service Quality Improvement and Resource Allocation
6. Energy and Utilities:
Grid Management and Predictive Maintenance
Energy Consumption Optimization
Equipment Monitoring and Failure Prediction
7. Transportation and Logistics:
Route Optimization and Fleet Management
Demand Forecasting and Supply Chain Visibility
Predictive Maintenance for Vehicles and Infrastructure
8. Government and Public Services:
Crime Pattern Analysis and Public Safety
Urban Planning and Traffic Management
Healthcare Policy and Resource Allocation
The widespread applications of data analytics across industries highlight its versatility and impact in optimizing processes, enhancing decision-making, and driving innovation in various sectors.
Comments