Essence of Descriptive Analytics in data analytics: Exploring the essentials in descriptive analytics, its main features, applications and techniques.
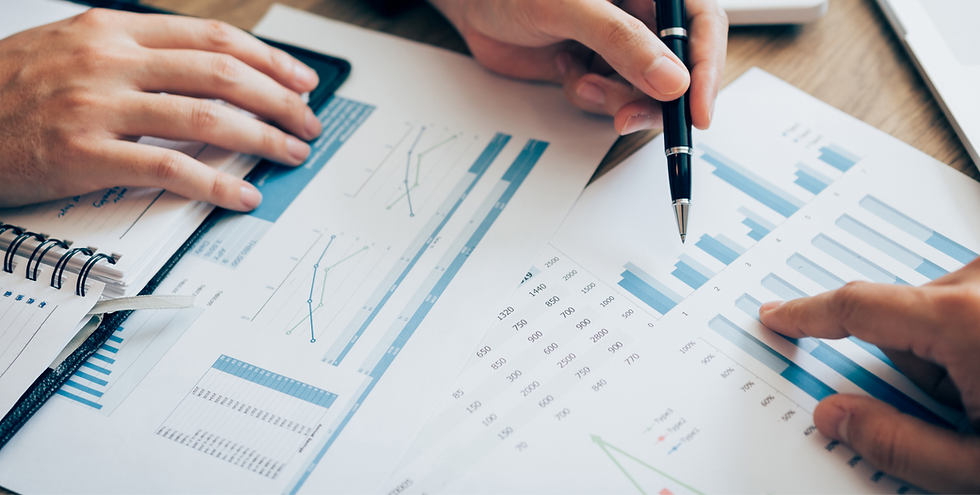
What is Descriptive Analytics?
Descriptive analytics is the foundational stage of data analytics that involves examining historical data to gain insights and understand patterns, trends, and relationships within the dataset. It focuses on summarizing and visualizing data to describe what has happened in the past and provides context for further analysis. This initial phase forms the basis for more advanced analytics, including predictive and prescriptive analytics. Descriptive analytics forms the groundwork for subsequent stages of data analysis. By providing a comprehensive understanding of historical data, it helps organizations make informed decisions, identify opportunities for improvement, and lay the foundation for more advanced analytics techniques.
Key Aspects of Descriptive Analytics:
Data Collection and Cleaning:
Descriptive analytics begins with data collection from various sources, followed by data cleaning to ensure accuracy and consistency. It involves handling missing values, outliers, and formatting issues.
Data Summarization and Aggregation:
Summarizing data using statistical measures like mean, median, mode, and standard deviation helps in understanding the central tendencies and distributions within the dataset. Aggregating data into categories or groups provides a high-level overview.
Visualization Techniques:
Visualization tools such as charts, graphs, histograms, and heatmaps help in presenting data visually. This aids in identifying trends, patterns, and outliers more intuitively.
Exploratory Data Analysis (EDA):
EDA techniques, like scatter plots, box plots, and correlation matrices, facilitate in-depth exploration of relationships between variables, uncovering insights that can guide further analysis.
Techniques and Methods in Descriptive Analytics
Descriptive analytics encompasses various techniques and methods to summarize, visualize, and understand historical data. Here's a detailed account of the techniques involved in descriptive analytics:
Measures of Central Tendency:
Mean: Mean is the average of the given numbers and is calculated by dividing the sum of given numbers by the total number of numbers.
Median: The median is the middle value in a set of data. First, organize and order the data from smallest to largest. To find the midpoint value, divide the number of observations by two. If there are an odd number of observations, round that number up, and the value in that position is the median.
Mode: A mode is defined as the value that has a higher frequency in a given set of values.
Measures of Dispersion:
Standard Deviation: Standard deviation is a statistic that measures the dispersion of a dataset relative to its mean and is calculated as the square root of the variance.
Variance: The term variance refers to a statistical measurement of the spread between numbers in a data set. More specifically, variance measures how far each number in the set is from the mean (average), and thus from every other number in the set.
Range: The range in statistics for a given data set is the difference between the highest and lowest values.
Graphical Representation:
Histograms: A histogram is a graph that shows the frequency of numerical data using rectangles. The height of a rectangle (the vertical axis) represents the distribution frequency of a variable (the amount, or how often that variable appears).
Bar Charts: A bar chart or bar graph is a chart or graph that presents categorical data with rectangular bars with heights or lengths proportional to the values that they represent. The bars can be plotted vertically or horizontally. A vertical bar chart is sometimes called a column chart.
Line Charts: These are a fundamental chart type generally used to show change in values across time.
Pie Charts: A pie chart is a type of graph representing data in a circular form, with each slice of the circle representing a fraction or proportionate part of the whole. All slices of the pie add up to make the whole equaling 100 percent and 360 degrees.
Scatter Plots: A scatter plot (aka scatter chart, scatter graph) uses dots to represent values for two different numeric variables. The position of each dot on the horizontal and vertical axis indicates values for an individual data point. Scatter plots are used to observe relationships between variables.
Bubble Charts: A bubble chart is primarily used to depict and show relationships between numeric variables. They are a great tool to establish the relationship between variables and examine relationships between key business indicators, such as cost, value and risk.
Box Plots: A boxplot is a standardized way of displaying the dataset based on the five-number summary: the minimum, the maximum, the sample median, and the first and third quartiles. Minimum (Q0 or 0th percentile): the lowest data point in the data set excluding any outliers.
Whisker Plots: A Box and Whisker Plot (or Box Plot) is a convenient way of visually displaying groups of numerical data through their quartiles.
Clustering and Segmentation Techniques:
K-Means Clustering: The method is a local search that iteratively attempts to relocate a sample into a different clusters long as this process improves the objective function.
Hierarchical Clustering: Hierarchical clustering is a popular method for grouping objects. It creates groups so that objects within a group are similar to each other and different from objects in other groups. Clusters are visually represented in a hierarchical tree called a dendrogram.
Other Related Techniques
Correlation Matrix: A correlation matrix is a statistical technique used to evaluate the relationship between two variables in a data set. The matrix is a table in which every cell contains a correlation coefficient, where 1 is considered a strong relationship between variables, 0 a neutral relationship and -1 a not strong relationship.
Frequency Tables: A frequency table shows the distribution of observations based on the options in a variable. Frequency tables are helpful to understand which options occur more or less often in the dataset.
Percentiles & Quartiles: Percentiles are a type of quantiles, obtained adopting a subdivision into 100 groups. The 25th percentile is also known as the first quartile (Q1), the 50th percentile as the median or second quartile (Q2), and the 75th percentile as the third quartile (Q3).
Cross-Tabulations: a cross-tabulation is a two- (or more) dimensional table that records the number (frequency) of respondents that have the specific characteristics described in the cells of the table. Cross-tabulation tables provide a wealth of information about the relationship between the variables.
Pivot Tables: A Pivot Table is an interactive way to quickly summarize large amounts of data.
Word Frequency Analysis: Word frequency analysis is the most basic and common method of qualitative text data analysis. It involves counting up mentions of a particular word or phrase as a means of understanding the dominant topics in a particular data set.
EDA: Exploratory Data Analysis (EDA) is an analysis approach that identifies general patterns in the data. These patterns include outliers and features of the data that might be unexpected. EDA is an important first step in any data analysis.
These techniques in descriptive analytics enable data analysts and decision-makers to explore, summarise, and interpret historical data effectively, facilitating insights and informed decision-making. Each technique serves a unique purpose in understanding and describing various aspects of the data.
Relationship between Descriptive
and Statistical Analysis
Descriptive analysis summarizes and describes the dataset, whereas statistical analysis extends beyond description to inferential and predictive analysis, leveraging mathematical models to make inferences or predictions about populations based on sample data. Both are integral parts of the data analysis process, with descriptive analysis laying the foundation for subsequent statistical and inferential analyses.
Complementary Roles: Descriptive analysis forms the groundwork for statistical analysis. Before applying statistical techniques, understanding the data's basic characteristics through descriptive analysis is crucial.
Informing Further Analysis: Descriptive statistics provide insights that guide the selection of appropriate statistical tests or models in subsequent analyses. For instance, identifying data distributions or outliers might prompt specific statistical approaches.
Iterative Process: Descriptive and statistical analyses often work iteratively. Descriptive analysis might reveal patterns or outliers that lead to hypothesis generation and testing through statistical methods, and insights from statistical analysis might prompt further exploration in descriptive analysis.
Applications of descriptive analytics
Descriptive analytics finds diverse applications across various industries and domains, serving as the bedrock for understanding historical data and gaining insights into past trends, patterns, and behaviours. Here's an in-depth exploration of its applications:
Business Performance Analysis:
Descriptive analytics is extensively used in businesses to analyze historical data related to sales, revenue, expenses, and operational metrics. It helps in assessing past performance, identifying growth areas, and understanding trends to make informed decisions. By examining key performance indicators (KPIs) over time, businesses can gauge their success, market share, and profitability.
Market Research and Consumer Behavior Analysis:
In market research, descriptive analytics plays a pivotal role in understanding consumer behavior, preferences, and market trends. It helps in segmenting customers based on demographics, purchase history, or geographic location. By analyzing historical data, businesses can identify target markets, tailor marketing strategies, and launch products or services that align with consumer preferences.
Healthcare and Public Health Planning:
In the healthcare sector, descriptive analytics aids in analyzing patient records, disease patterns, treatment outcomes, and healthcare utilization. It assists in identifying prevalent diseases, demographic trends, and areas with specific healthcare needs. Public health planners leverage this data to allocate resources, plan interventions, and develop preventive strategies for communities.
Operational Efficiency and Process Improvement:
Descriptive analytics is instrumental in monitoring and optimizing operational processes across industries. In manufacturing, it helps in analyzing production data to identify bottlenecks, optimize workflows, and enhance efficiency. In supply chain management, it aids in tracking inventory levels, transportation routes, and delivery performance to streamline operations.
Financial Analysis and Risk Management:
Financial institutions utilize descriptive analytics to analyze historical financial data, market trends, and risk factors. It helps in assessing investment portfolios, evaluating credit risk, and identifying potential financial threats. By studying past financial performance, organizations can mitigate risks, improve investment strategies, and ensure regulatory compliance.
Fraud Detection and Security:
In the realm of security and fraud detection, descriptive analytics examines historical patterns and anomalies in data to detect potential fraudulent activities. It aids in identifying suspicious behavior, irregular transactions, or cybersecurity threats by analyzing historical data patterns and deviations.
Sports Analytics and Performance Tracking:
In sports, descriptive analytics is used to track player performance, analyze game statistics, and evaluate team strategies. It helps in assessing player performance over time, identifying strengths and weaknesses, and making informed decisions regarding team strategies and training programs.
Educational Institutions and Student Performance:
Educational institutions leverage descriptive analytics to analyze student performance, attendance records, and learning outcomes. It helps in identifying areas for improvement, evaluating teaching methods, and implementing interventions to enhance student success.
Descriptive analytics, through its diverse applications, provides valuable insights derived from historical data, enabling organizations across industries to make informed decisions, optimize processes, and improve overall performance. Descriptive analytics acts as a stepping stone in the data analytics journey, enabling the transformation of raw data into meaningful insights that drive informed decision-making and business strategies.
Comments