Diagnostic Analytics: The Crucial Role of Diagnostic Analytics and involved techniques in Deciphering Complexities for Strategic Decision-Making and unearthing insights from historic datasets.
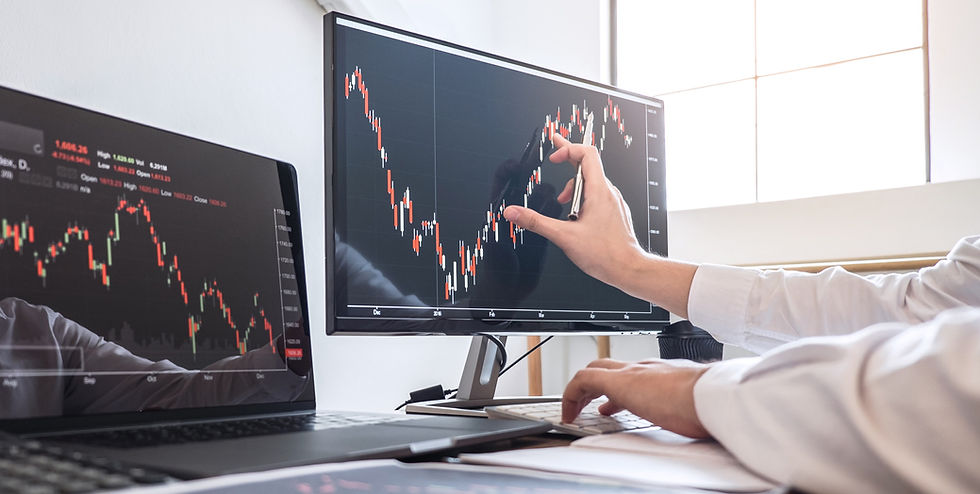
What is Diagnostic Analytics?
Diagnostic analytics is a branch of analytics that focuses on examining data to understand why certain events or outcomes occurred within a specific context. It involves deep analysis of historical data to identify patterns, correlations, or anomalies that help explain past performance or occurrences. Diagnostic analytics is a facet of data analysis that delves into the depths of historical data to unravel the causes behind specific events or outcomes. It's akin to investigating the 'why' behind the 'what.' This branch of analytics doesn't just highlight trends or patterns; it goes further, aiming to unearth the reasons and factors driving those patterns. By scrutinizing correlations, anomalies, or relationships within datasets, diagnostic analytics aims to pinpoint the root causes of successes, failures, anomalies, or shifts in trends. It employs various methods such as statistical analysis, regression, and anomaly detection to dissect data, providing insights that help explain past performances or occurrences. This kind of analytics is invaluable across industries, aiding businesses in understanding the drivers of their success, unraveling the mysteries behind failures, and paving the way for informed decision-making based on robust historical evidence.
Diagnostic Analytics - Techniques
Diagnostic analytics involves a range of techniques aimed at dissecting data to uncover the underlying causes behind specific outcomes or events. Diagnostic analytics, through these techniques, enables organizations to unravel complexities within their data, providing valuable insights into past occurrences and facilitating better decision-making for the future. Here are some key techniques used in diagnostic analytics:
1. Descriptive Statistics:
Descriptive statistics are the bedrock of data analysis, offering a succinct summary of the key features within a dataset. These statistical measures, ranging from central tendencies like mean, median, and mode to dispersion indicators such as variance and standard deviation, provide a comprehensive snapshot of the dataset's characteristics. They unveil the distribution's shape, whether it's symmetric or skewed, and help discern the presence of outliers or unusual patterns. Through percentiles and measures like quartiles and ranges, descriptive statistics offer insights into data spread and variability. Visual representations like histograms further aid in comprehending the frequency distribution. Altogether, descriptive statistics serve as a compass, guiding analysts and researchers in understanding, interpreting, and communicating the essence of the data to make informed decisions or draw meaningful conclusions.
Mean, Median, Mode: These measures provide central tendencies, giving an idea about the typical value within a dataset.
Variance and Standard Deviation: They depict the dispersion or spread of data points around the mean, showing how much individual data points differ from the average.
2. Data Visualization:
Data visualization is the art of presenting complex information and datasets in a visually appealing and comprehensible format. It goes beyond mere charts and graphs; it's a language that transforms raw data into insightful narratives and patterns. Through various visual tools like graphs, charts, maps, and infographics, data visualization unveils trends, relationships, and outliers that might not be immediately evident in raw data. It enables quick comprehension, facilitating better decision-making and communication. By leveraging color, size, shapes, and interactive elements, data visualization engages audiences, making data more accessible and compelling. It bridges the gap between data analysis and understanding, empowering individuals across diverse fields—from business analytics to scientific research—to derive actionable insights and tell compelling stories from the wealth of available information.
Charts and Graphs: Visual representations such as bar graphs, line charts, histograms, or scatter plots help in understanding trends, patterns, and outliers within data.
Dashboards: Interactive and dynamic displays of key metrics and trends, offering a comprehensive view of multiple data points.
3. Correlation Analysis:
Correlation analysis is a powerful statistical method used to examine the relationship between two or more variables in a dataset. It measures the strength and direction of the linear association between these variables, indicating how changes in one variable might correspond to changes in another. The correlation coefficient, typically represented by the Pearson correlation coefficient, ranges from -1 to 1: a value close to 1 suggests a strong positive linear relationship, while a value close to -1 indicates a strong negative linear relationship. A value around 0 implies a weak or no linear relationship. Correlation analysis aids in understanding connections between variables, guiding decision-making processes and predictive models. However, it's crucial to note that correlation doesn’t imply causation—just because variables are correlated doesn't mean that one causes the other. It serves as a vital tool in many fields, including economics, social sciences, and data analytics, helping analysts and researchers to uncover meaningful associations within datasets.
4. Regression Analysis:
Regression analysis is a fundamental statistical technique used to explore the relationship between a dependent variable and one or more independent variables. Its primary goal is to understand how changes in the independent variables are associated with changes in the dependent variable. By fitting a regression model, often represented as a line or curve, to the data points, it allows for prediction, explanation, and inference. There are various types of regression models, such as linear regression, logistic regression, and polynomial regression, each suitable for different types of data and relationships. Regression analysis provides insights into the strength and direction of the relationships between variables, enabling predictions of future outcomes based on historical data. It's a pivotal tool in fields like machine learning, data analytics, economics, finance, healthcare, and social sciences, aiding in decision-making, hypothesis testing, and understanding the complex interplay among variables within a system. However, it's important to interpret regression results cautiously, considering factors like model assumptions, potential biases, and the limitations of the data.
5. Anomaly Detection:
Anomaly detection is a crucial technique within data analysis focused on identifying patterns, events, or observations that deviate significantly from the expected or normal behavior within a dataset. Its primary objective is to pinpoint rare occurrences that stand out as anomalies, potentially indicating errors, fraud, or valuable insights. Various methods, including statistical approaches, machine learning algorithms, and pattern recognition techniques, are employed to detect these anomalies. Unsupervised learning methods like clustering or density estimation, as well as supervised methods using labeled data, are often utilized for this purpose. Anomaly detection finds applications across diverse fields such as cybersecurity, finance, healthcare, and manufacturing, aiding in fraud detection, fault diagnosis, quality control, and predictive maintenance. However, the challenge lies in differentiating true anomalies from normal variations and noise while adapting to evolving patterns in complex datasets, making anomaly detection an ongoing and adaptive process crucial for maintaining data integrity and making informed decisions.
6. Cohort Analysis:
Cohort analysis is a powerful method used in statistics and business analytics to understand and track the behavior and characteristics of specific groups, or cohorts, over time. By grouping individuals who share a common characteristic or experience within a defined timeframe, such as customers who signed up during a particular month or employees hired in a specific year, cohort analysis enables a detailed examination of their shared journey or patterns. It allows for the comparison of how different cohorts behave or perform, unveiling insights into trends, changes, or specific outcomes unique to each cohort. This analysis is pivotal in various fields, notably in marketing to assess customer lifetime value, retention rates, and product performance, as well as in healthcare to study patient outcomes based on treatment cohorts. Cohort analysis helps businesses and researchers make data-driven decisions by identifying trends and understanding the impact of certain actions or events on different groups over time.
Grouping Data: Segregating data into cohorts based on shared characteristics (e.g., age groups, customer segments) to analyze trends or behaviors within specific groups.
7. Hypothesis Testing:
Hypothesis testing serves as the backbone of statistical inference, offering a systematic approach to validate or refute claims about population parameters using sample data. It revolves around assessing the strength of evidence against the null hypothesis, which typically represents the status quo or absence of an effect. The process involves setting up a null hypothesis (H0) and an alternative hypothesis (H1), selecting a significance level to determine the threshold for accepting or rejecting the null hypothesis, choosing an appropriate statistical test, collecting data, and drawing conclusions based on the test results. The significance level, often denoted as α, determines the probability of making a Type I error—rejecting a true null hypothesis. Statistical tests generate a test statistic, compared to a critical value to decide whether to reject the null hypothesis. If the test statistic falls into the critical region, beyond the critical value, the null hypothesis is rejected in favor of the alternative. Hypothesis testing finds extensive application in research, quality control, medical trials, and business analytics, providing a structured approach to make evidence-based decisions and draw meaningful conclusions about the characteristics of populations or the effectiveness of interventions. Its robust framework ensures that conclusions drawn from sample data are grounded in statistical evidence, offering a reliable means to assess claims and drive informed decision-making.
T-Tests, Chi-Square Tests: Statistical techniques used to test hypotheses about the relationships or differences between variables within a dataset.
8. Root Cause Analysis:
Root cause analysis (RCA) is a systematic method used to identify the underlying reason(s) responsible for a problem or an occurrence, aiming to address the core issue rather than its symptoms. It involves a structured approach that investigates events, incidents, or issues to understand the chain of causes leading to the observed outcome. The primary goal is to prevent the problem from recurring by addressing its fundamental causes. RCA is employed across various industries, including healthcare, manufacturing, aviation, and engineering, as a proactive approach to problem-solving and continuous improvement. By focusing on addressing the fundamental reasons behind issues, RCA aims to prevent future occurrences, enhance processes, and promote a culture of learning and improvement within organizations.
Fishbone Diagrams, 5 Whys: Qualitative techniques aimed at systematically identifying the underlying causes of issues or problems within a system.
9. Time Series Analysis:
Time series analysis is a statistical method used to analyze sequential data points collected and recorded over regular intervals of time. It focuses on understanding, modeling, and forecasting patterns, trends, and behaviors within the data to extract meaningful insights. Time series analysis finds extensive applications in various fields, including finance for stock market predictions, economics for GDP forecasting, weather forecasting, demand forecasting in supply chain management, and analyzing trends in health metrics. Its insights aid in decision-making processes, strategic planning, and understanding the dynamics of evolving systems over time.
Future Advancements and Applications
The future of diagnostic analytics seems promising, marked by advancements in technology, data accessibility, and more sophisticated analytical tools. Here are some potential trends and developments:
AI and Machine Learning Integration: Increased utilization of artificial intelligence (AI) and machine learning algorithms will enhance diagnostic analytics capabilities. These technologies will enable more automated and precise analysis of complex datasets, offering deeper insights and faster decision-making.
Real-Time Diagnostic Insights: The focus will shift towards real-time analytics, allowing organizations to identify and respond to issues as they occur. This will involve the integration of streaming data sources and the development of algorithms capable of processing data in real-time.
Predictive Diagnostic Analytics: Diagnostic analytics will increasingly incorporate predictive elements, allowing for proactive identification of potential issues before they occur. This predictive capability will help organizations prevent problems, optimize processes, and reduce risks.
Advanced Visualization and Interactivity: Visualization tools will become more sophisticated, providing interactive and intuitive interfaces for users to explore and understand data. Augmented and virtual reality might play a role in creating immersive data experiences for better analysis.
Ethical and Privacy Considerations: With the growing emphasis on data privacy and ethics, future developments in diagnostic analytics will need to address concerns surrounding data security, privacy regulations, and ethical use of data.
Edge Analytics: Analytical capabilities will move closer to the data source with the advancement of edge computing. This trend will facilitate faster data processing, reduce latency, and enable analysis in environments with limited connectivity.
Cross-Domain Integration: There will be a move towards integrating data from multiple domains and sources. This interdisciplinary approach will enable a more comprehensive understanding of complex problems and foster innovation through the synthesis of diverse datasets.
Overall, the future of diagnostic analytics holds promise for more efficient, accurate, and timely insights. As technology evolves and data processing capabilities advance, organizations will leverage these developments to gain a deeper understanding of their operations, make better decisions, and drive innovation.
Comments