Exploring Generative AI: The Creative Power of Machines
- Samul Black
- Feb 11, 2024
- 7 min read
Generative Artificial Intelligence (AI) represents a remarkable fusion of technology and creativity, enabling machines to produce original content, such as images, text, music, and even videos. From generating realistic human faces to composing symphonies, generative AI algorithms have made significant strides in mimicking and augmenting human creativity. In this article, we delve into the world of generative AI, examining its impact, significance, involved algorithms, applications, challenges, and the ethical considerations surrounding its development and use.
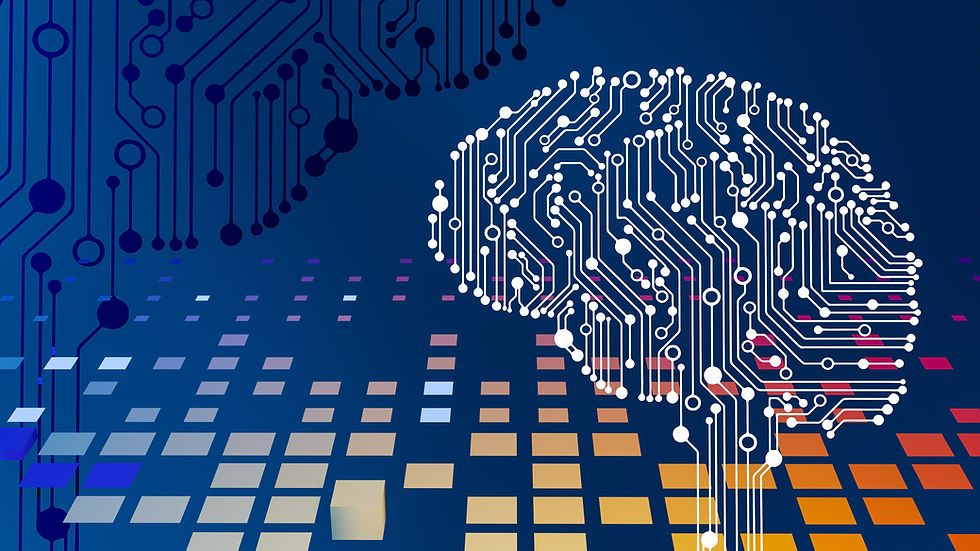
What is Generative Artificial Intelligence (AI)?
Generative Artificial Intelligence (AI) is a transformative subset of machine learning that enables algorithms to create new content resembling data they've been trained on. Unlike traditional AI models that focus on recognizing patterns within existing datasets, generative AI ventures into the realm of creativity by producing novel outputs. This innovation has led to remarkable advancements in various domains, including image generation, text synthesis, music composition, and even drug discovery. At its core, generative AI operates on complex probabilistic models and neural networks, such as Generative Adversarial Networks (GANs) and Variational Autoencoders (VAEs), which learn to generate data distributions and capture intricate patterns within datasets.The evolution of generative AI has been marked by significant breakthroughs and rapid progress, driven by advancements in deep learning architectures, computational power, and access to vast amounts of data. Early generative models struggled to produce realistic outputs and often generated artifacts that were easily distinguishable from authentic content. However, with the advent of techniques like GANs, which pit two neural networks against each other in a competitive manner—one generating content and the other discerning between real and fake—the quality of generated outputs has improved dramatically. Furthermore, innovations in natural language processing (NLP) have led to the development of language models capable of generating coherent and contextually relevant text, opening new frontiers in content creation and communication. As generative AI continues to evolve, it promises to redefine how we interact with technology and unleash unprecedented levels of creativity and innovation.
Impact and Significance of Generative Artificial Intelligence (AI)
Generative AI has emerged as a transformative force in today's world, reshaping industries, unlocking new possibilities, and redefining the boundaries of human-machine interaction. One of the most significant impacts of generative AI lies in its ability to democratize creativity, enabling individuals and businesses alike to generate high-quality content across various domains. Whether it's generating realistic images, composing original music, or crafting compelling narratives, generative AI empowers creators to express themselves in unprecedented ways, fueling innovation and fostering a vibrant digital ecosystem. This democratization of creativity not only accelerates the pace of content creation but also fosters inclusivity by providing opportunities for diverse voices and perspectives to be heard and amplified. Moreover, generative AI holds immense potential to address complex societal challenges and drive positive social change. From advancing healthcare through drug discovery and medical image analysis to mitigating environmental issues through predictive modeling and resource optimization, generative AI offers innovative solutions to some of the most pressing problems facing humanity. By harnessing the power of data-driven insights and predictive analytics, generative AI enables more informed decision-making, enhances efficiency in resource allocation, and facilitates the development of targeted interventions and policies. As such, the significance of generative AI in today's world extends beyond its technological prowess, encompassing its capacity to catalyze progress and contribute to the betterment of society as a whole.
Algorithms for Building Generativie Artificially Intelligent Models
Generative Artificial Intelligence (AI) employs various algorithms and techniques to generate new content across different domains. Some of the most commonly used algorithms include:
Generative Adversarial Networks (GANs): GANs consist of two neural networks—the generator and the discriminator—competing against each other in a game-like setup. The generator generates synthetic data samples, while the discriminator tries to distinguish between real and generated samples. Through adversarial training, GANs learn to generate increasingly realistic data instances, such as images, by iteratively improving the generator's ability to deceive the discriminator.
Variational Autoencoders (VAEs): VAEs are a type of neural network architecture used for unsupervised learning and data generation. VAEs consist of an encoder network that maps input data to a latent space and a decoder network that reconstructs the input data from samples drawn from the latent space. By learning a probabilistic distribution over the latent space, VAEs can generate new data samples by sampling from the learned distribution and decoding them through the decoder network.
Autoregressive Models: Autoregressive models, such as Recurrent Neural Networks (RNNs) and Transformers, generate data sequentially, one element at a time, based on previous elements in the sequence. These models learn the conditional probability distribution of the next element given the previous elements in the sequence. Autoregressive models are commonly used for generating sequences of text, music, and time-series data.
Boltzmann Machines: Boltzmann Machines are a type of stochastic recurrent neural network that models the joint probability distribution of binary random variables. These models learn to generate new data samples by sampling from the learned distribution, capturing complex dependencies and patterns in the data. Boltzmann Machines are particularly useful for modeling high-dimensional data with intricate correlations.
These algorithms represent a diverse set of approaches to generative modeling, each with its strengths and limitations. By leveraging these techniques, generative AI enables machines to generate diverse and realistic content across various domains, revolutionizing creativity, innovation, and problem-solving.
Applications of Generative Artificial Intelligence
Generative AI finds applications across diverse domains, each harnessing its creative potential to solve unique challenges and enhance various aspects of human life. Here are some of the key applications:
Image Generation and Editing: Generative AI has revolutionized the field of computer vision by enabling the generation of high-quality, photorealistic images. Applications range from generating synthetic training data for machine learning models to creating virtual environments for gaming and simulation. Moreover, generative models like Generative Adversarial Networks (GANs) have empowered artists and designers with tools for image editing and manipulation, allowing for the creation of visually stunning graphics, special effects, and digital artwork. Furthermore, in fields such as architecture and interior design, generative AI facilitates the rapid prototyping and visualization of design concepts, accelerating the creative process and enabling stakeholders to explore various design possibilities before committing to a final product.
Text Generation and Natural Language Processing (NLP): Generative AI has transformed the way we interact with language, enabling the generation of coherent and contextually relevant text across various applications. Language models like OpenAI's GPT (Generative Pre-trained Transformer) have demonstrated remarkable capabilities in tasks such as text completion, summarization, and translation. These models have implications for content generation, storytelling, and language understanding, powering virtual assistants, chatbots, and recommendation systems. Moreover, in the field of journalism and content creation, generative AI offers tools for generating news articles, product descriptions, and marketing copy, augmenting human creativity and productivity in the digital age.
Music Composition and Generation: Generative AI has unlocked new possibilities in music composition and generation, enabling the creation of original compositions across diverse genres and styles. Through techniques such as recurrent neural networks (RNNs) and variational autoencoders (VAEs), generative models can learn the underlying structure and patterns of musical compositions and generate new pieces based on learned representations. These models have implications for music production, personalized playlists, and interactive music experiences. Additionally, generative AI fosters collaboration between humans and machines in the creative process, empowering musicians, composers, and artists with tools for inspiration, experimentation, and exploration in the realm of music.
Drug Discovery and Molecular Design: Generative AI plays a vital role in accelerating drug discovery and development processes by generating novel chemical compounds with desired properties. Through techniques such as deep reinforcement learning and molecular optimization, generative models can explore vast chemical spaces, identify promising drug candidates, and optimize molecular structures for specific targets. These models have implications for pharmaceutical research, personalized medicine, and drug repurposing, offering potential solutions to complex diseases and medical challenges. Moreover, generative AI enables the design of new materials with tailored properties, driving innovation in fields such as materials science, nanotechnology, and renewable energy.
Generative AI continues to push the boundaries of innovation and creativity across various domains, empowering individuals and organizations with tools for exploration, discovery, and expression in the digital age. As research and development efforts in generative modeling advance, the potential for transformative applications in fields such as healthcare, entertainment, and education is vast, paving the way for a future where machines collaborate with humans to achieve remarkable feats of creativity and ingenuity.
Challenges and Limitations
Despite its transformative potential, generative AI faces several challenges and limitations that warrant consideration:
Bias and Fairness: Generative models can inadvertently perpetuate biases present in the training data, leading to biased outputs. Addressing issues of fairness and equity in generative AI systems requires careful data curation and algorithmic transparency.
Ethical Concerns: The proliferation of generative AI raises ethical concerns surrounding issues such as copyright infringement, misinformation dissemination, and the creation of deepfakes—synthetic media designed to deceive or manipulate viewers.
Control and Accountability: As generative AI becomes more sophisticated, ensuring control and accountability over generated content becomes increasingly challenging. Establishing mechanisms for content validation and attribution is essential to mitigate potential misuse.
Future Directions: Despite the challenges, the future of generative AI holds immense promise, with ongoing research and development efforts focusing on addressing its limitations and expanding its capabilities. Some future directions include:
Interdisciplinary Collaboration: Collaborations between AI researchers, domain experts, ethicists, and policymakers are essential to develop responsible and ethical applications of generative AI.
Explainable AI: Enhancing the interpretability of generative AI algorithms can facilitate greater transparency and trust in their outputs, enabling users to understand how and why certain content is generated.
Robustness and Security: Developing robust defenses against adversarial attacks and ensuring the security of generative AI systems will be crucial for safeguarding against potential misuse and exploitation.
Human-AI Collaboration: Embracing a collaborative approach where humans and AI systems work together synergistically can unlock new opportunities for creativity, innovation, and societal impact.
Conclusion: Generative AI represents a paradigm shift in artificial intelligence, empowering machines to exhibit creativity and imagination previously reserved for human creators. While its potential is vast, navigating the ethical, societal, and technical challenges associated with generative AI will require concerted efforts from researchers, policymakers, and society at large. By fostering responsible development and deployment practices, we can harness the creative power of generative AI to drive positive change and innovation across diverse domains.
Commentaires