Generative AI, a subset of artificial intelligence, has been making waves across various industries, from art and entertainment to science and technology. Unlike traditional AI, which focuses on analyzing data and making predictions, generative AI is designed to create new content, such as text, images, music, and even entire virtual worlds. This capability to generate novel and creative outputs has far-reaching implications, transforming the way we think about creativity, innovation, and problem-solving. In this blog, we will explore what generative AI is, how it works, its key applications, the challenges it presents, and its future potential.
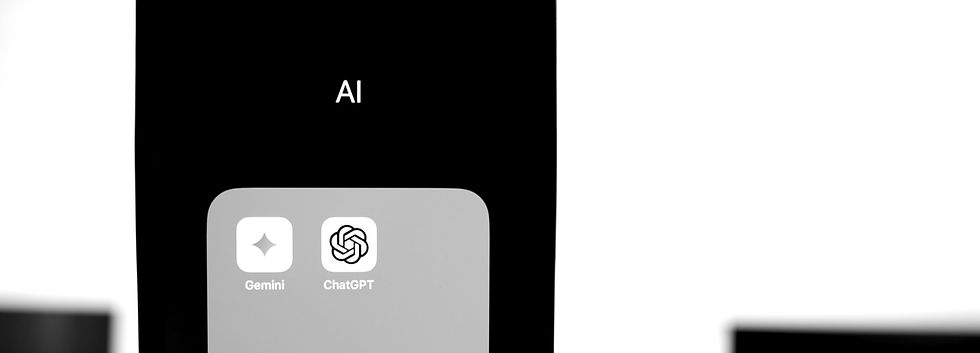
What is Generative AI?
Generative AI refers to algorithms and models that can produce new data or content that resembles the training data they were fed. This is in contrast to discriminative models, which focus on classifying or predicting outcomes based on input data. Generative AI models learn patterns and structures from existing data and use this knowledge to generate new, original outputs.
Some of the most well-known generative AI models include Generative Adversarial Networks (GANs), Variational Autoencoders (VAEs), and Transformer-based models like GPT (Generative Pre-trained Transformer). These models have been used to create everything from realistic images of non-existent people to coherent and contextually appropriate text.
How Does Generative AI Work?
Generative AI models typically work by learning from large datasets and then using this learned information to generate new outputs. Let’s take a closer look at some of the key types of generative models:
1. Generative Adversarial Networks (GANs)
GANs consist of two neural networks—a generator and a discriminator—that are trained together. The generator creates fake data, such as images, while the discriminator evaluates how close the generated data is to the real data. The generator’s goal is to produce data that is indistinguishable from real data, while the discriminator tries to identify the fake data. Through this adversarial process, GANs become highly effective at generating realistic content.
2. Variational Autoencoders (VAEs)
VAEs are another type of generative model that learns to encode data into a compressed format (latent space) and then decode it back into original or new forms. VAEs are particularly useful for generating variations of a given dataset, such as different styles of images or different versions of a piece of music.
3. Transformer-based Models
Transformer models, like GPT, have revolutionized the field of natural language processing (NLP). These models are trained on vast amounts of text data and can generate coherent and contextually appropriate text by predicting the next word in a sequence. This capability has led to the development of AI systems that can write essays, create poetry, and even engage in conversations that mimic human interaction.
Key Applications of Generative AI
Generative AI has a wide range of applications across various domains. Here are some of the most notable:
1. Art and Design
Generative AI is being used to create stunning works of art, generate design ideas, and even assist in architectural planning. Artists and designers use AI to explore new creative possibilities, from generating unique patterns and textures to creating entire digital artworks.
2. Content Creation
In the world of content creation, generative AI is being used to write articles, create marketing copy, and generate social media posts. AI-generated content can be used to supplement human creativity, providing ideas, drafts, and even fully-formed pieces of content that can be fine-tuned by human editors.
3. Music Composition
Generative AI models are capable of composing music in a variety of genres, mimicking the styles of famous composers, or creating entirely new musical pieces. This technology is being used by musicians and producers to experiment with new sounds and generate background music for films, video games, and other media.
4. Video Game Development
In video game development, generative AI is being used to create realistic characters, landscapes, and scenarios. AI can generate entire levels, populate worlds with dynamic and adaptive content, and even create non-playable characters (NPCs) with believable behaviors and dialogue.
5. Healthcare and Drug Discovery
Generative AI is also making a significant impact in healthcare, particularly in drug discovery. By generating molecular structures and simulating their interactions with biological systems, AI can help identify new drug candidates and accelerate the development of treatments.
6. Synthetic Data Generation
In machine learning, generative AI is used to create synthetic data, which can be invaluable for training models when real-world data is scarce or sensitive. Synthetic data can help improve model performance, enhance privacy, and reduce biases in AI systems.
Challenges of Generative AI
Despite its vast potential, generative AI also presents several challenges:
Ethical Concerns: Generative AI raises ethical issues, particularly around the creation of deepfakes—realistic but fake images, videos, or audio recordings that can be used to spread misinformation or impersonate individuals. The ability to generate highly convincing content has led to concerns about privacy, consent, and the potential for misuse.
Quality Control: While generative AI can produce impressive results, the quality of the generated content can vary, and it may not always meet human standards for accuracy, coherence, or creativity. Ensuring that AI-generated content is reliable and trustworthy remains a significant challenge.
Intellectual Property: The use of AI to generate content raises questions about ownership and intellectual property rights. For example, if an AI generates a piece of music or art, who owns the rights to that creation? These legal and ethical questions are still being debated and will require careful consideration as the technology advances.
Bias and Fairness: Generative AI models learn from existing data, which can include biases present in the training data. If not carefully managed, these biases can be reflected in the AI’s output, leading to unfair or discriminatory results.
The Future of Generative AI
The future of generative AI is incredibly promising, with advancements in technology likely to address many of the current challenges and unlock new possibilities. As AI models become more sophisticated, we can expect to see even more realistic and creative outputs, blurring the lines between human and machine-generated content.
One exciting area of development is the integration of generative AI with other technologies, such as augmented reality (AR) and virtual reality (VR). This could lead to the creation of immersive experiences that are entirely AI-generated, offering new ways to interact with digital content.
Additionally, as AI becomes more accessible, we may see a democratization of creativity, where individuals without formal training in art, music, or writing can use AI tools to create professional-quality content. This could lead to a surge in creative expression and innovation, as more people are empowered to explore their ideas and bring them to life.
Conclusion
Generative AI is revolutionizing the way we think about creativity and innovation. By enabling machines to generate new and original content, AI is opening up new possibilities in art, design, content creation, and beyond. While there are challenges to overcome, the potential of generative AI to enhance human creativity, solve complex problems, and drive innovation is undeniable. As we continue to explore the capabilities of this technology, generative AI is set to play a pivotal role in shaping the future of creativity and the digital landscape.
Comentarios