The field of molecular chemistry is undergoing a transformation, driven by the integration of Machine Learning (ML) and Artificial Intelligence (AI). Traditionally, the study of molecules—their structures, properties, and interactions—has relied on experimental techniques and theoretical models. While these approaches have been successful, they are often time-consuming and resource-intensive. The advent of ML and AI is revolutionizing molecular chemistry by enabling faster, more accurate predictions, automating complex tasks, and uncovering new insights that were previously beyond reach. This blog explores how ML and AI are reshaping molecular chemistry, driving innovation, and accelerating discoveries in areas like drug design, material science, and environmental chemistry.
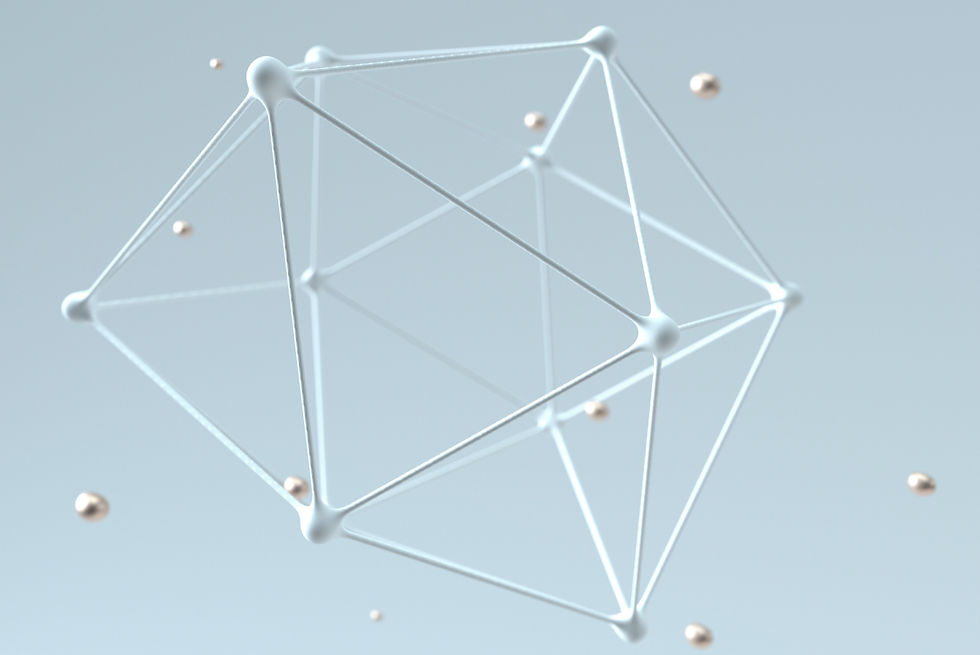
The Role of Machine Learning in Molecular Chemistry
Machine Learning in molecular chemistry involves training algorithms on vast datasets of molecular structures and properties. These algorithms learn patterns and relationships that can then be used to predict the behavior of new, unseen molecules. Some key applications include:
Molecular Property Prediction: ML models can predict properties such as solubility, toxicity, and reactivity based on a molecule's structure. This is particularly valuable in drug discovery, where predicting a compound's biological activity can significantly reduce the time and cost of developing new medications.
Molecular Docking and Binding Affinity: In drug design, understanding how a drug molecule binds to its target protein is crucial. ML algorithms can predict the binding affinity between molecules and proteins, guiding the selection of potential drug candidates with higher efficacy.
Chemical Reaction Prediction: ML models are used to predict the outcomes of chemical reactions, helping chemists design synthetic routes for new compounds. This reduces the need for trial-and-error experimentation, speeding up the development of new materials and drugs.
Material Design: In material science, ML is used to design new materials with desired properties, such as superconductors, catalysts, or polymers. By learning from existing materials, ML algorithms can suggest novel combinations of elements and structures that are likely to exhibit specific behaviors.
The Impact of Artificial Intelligence on Molecular Chemistry
Artificial Intelligence goes beyond traditional ML by enabling more advanced analysis and decision-making in molecular chemistry. AI systems can process complex, multi-dimensional data, identify hidden patterns, and optimize chemical processes in ways that are not possible with conventional methods. Here’s how AI is making an impact:
Automated Drug Discovery: AI-driven platforms can automate the drug discovery process, from initial screening to lead optimization. These systems analyze vast chemical libraries, predict drug-target interactions, and suggest modifications to improve efficacy and reduce side effects. This approach is significantly faster than traditional methods, potentially leading to the discovery of new drugs in record time.
Generative Models for Molecule Design: AI generative models, such as generative adversarial networks (GANs) and variational autoencoders (VAEs), can create entirely new molecular structures. By training on existing molecules, these models generate novel compounds with specific properties, opening up new possibilities for drug development and material science.
Quantum Chemistry Simulations: AI is being used to accelerate quantum chemistry simulations, which are essential for understanding the electronic properties of molecules. By approximating complex quantum calculations, AI models enable faster and more accurate simulations, aiding in the design of molecules with tailored properties.
Environmental Chemistry: AI is playing a crucial role in environmental chemistry by predicting the behavior of pollutants, optimizing processes for environmental remediation, and designing sustainable materials. AI models can analyze large datasets of environmental interactions, helping scientists develop solutions for pressing environmental challenges.
Real-World Applications of Machine Learning (ML) and Artificial Intelligence (AI) in Molecular Chemistry
Machine Learning (ML) and Artificial Intelligence (AI) are driving significant advancements in molecular chemistry with applications that are transforming industries. In drug design, ML and AI expedite the discovery of new medications by predicting molecular properties and drug-target interactions, reducing the time and cost of development. In material science, these technologies enable the creation of novel materials with tailored properties, such as more efficient catalysts or advanced polymers. AI also plays a crucial role in environmental chemistry, where it helps predict pollutant behavior, optimize remediation processes, and design sustainable materials. These real-world applications showcase the profound impact of ML and AI on accelerating innovation and solving complex challenges in molecular chemistry. The combination of ML and AI in molecular chemistry has led to several groundbreaking applications:
Drug Design and Development: Pharmaceutical companies are increasingly using ML and AI to streamline the drug discovery process. For example, AI models have been used to identify promising drug candidates for diseases such as cancer, Alzheimer’s, and COVID-19. These models help prioritize compounds that are most likely to succeed in clinical trials, reducing the time and cost of bringing new drugs to market.
Material Innovation: AI-driven material design is leading to the discovery of new materials with applications in energy storage, electronics, and nanotechnology. For instance, AI has been used to design novel catalysts for sustainable chemical reactions, as well as new polymers with enhanced properties for industrial applications.
Environmental Monitoring and Remediation: In environmental chemistry, AI is used to predict the fate of pollutants in ecosystems, optimize water treatment processes, and design materials for capturing and neutralizing contaminants. This has significant implications for addressing environmental challenges such as pollution and climate change.
Personalized Medicine: By analyzing the interactions between molecules and biological systems, AI is paving the way for personalized medicine. AI models can predict how individual patients will respond to specific drugs, allowing for the development of tailored treatment plans that maximize efficacy and minimize adverse effects.
Challenges and Future Directions
While the integration of ML and AI in molecular chemistry holds great promise, it also presents challenges. The quality and availability of data are critical, as ML models require large, diverse datasets to perform accurately. Additionally, the interpretability of AI models is an ongoing concern, as it is often difficult to understand how these models arrive at their predictions. Ensuring the ethical use of AI in chemistry, particularly in areas like drug design, is also crucial.
Looking forward, the continued development of AI techniques, such as explainable AI and transfer learning, will likely address some of these challenges. Moreover, the integration of AI with other advanced technologies, such as quantum computing, could further enhance the capabilities of molecular chemistry, leading to even more groundbreaking discoveries.
Conclusion
Machine Learning and Artificial Intelligence are revolutionizing molecular chemistry, enabling faster, more accurate predictions and opening up new avenues for discovery. From drug design and material innovation to environmental chemistry and personalized medicine, the impact of these technologies is profound and far-reaching. As ML and AI continue to evolve, their role in molecular chemistry will only grow, driving innovation and solving some of the most pressing challenges in science and industry. The future of molecular chemistry is bright, with ML and AI leading the way to a new era of discovery and advancement.
Comments