Unveiling Sentiments: The Power and Applications of Sentiment Analysis in the Digital Era
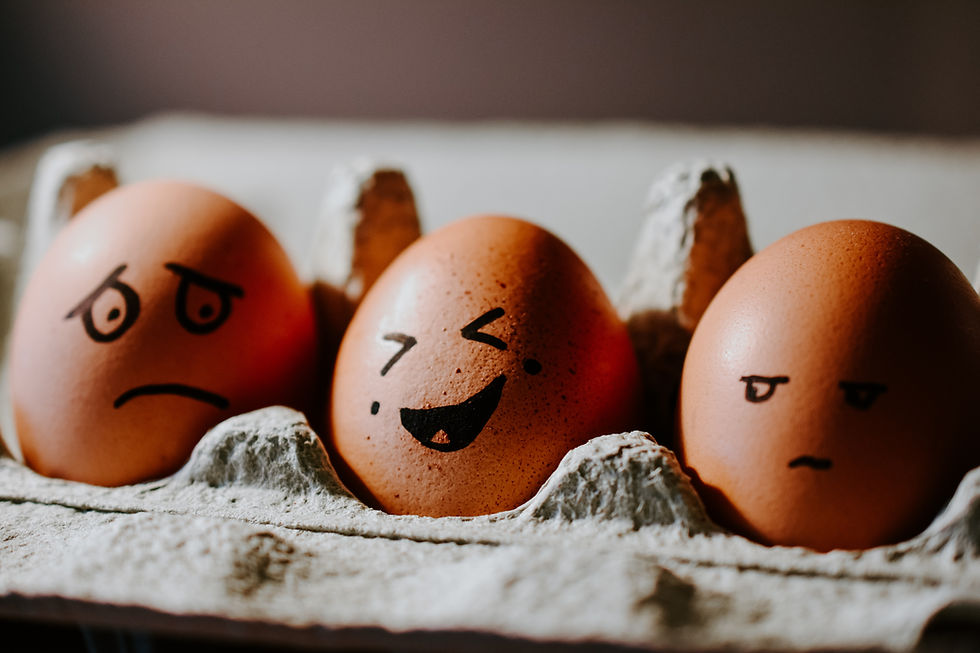
What is Sentiment Analysis?
In an era dominated by vast amounts of unstructured textual data, understanding human emotions and opinions is paramount. Sentiment analysis, a powerful application of natural language processing (NLP), emerges as a pivotal tool in deciphering the underlying sentiments expressed within texts, unlocking invaluable insights across diverse domains. At its core, sentiment analysis is a computational technique designed to discern and categorize sentiments—be they positive, negative, or neutral—embedded within written or spoken language. Through sophisticated algorithms and machine learning models, this process enables the automated extraction of sentiments from a multitude of sources, spanning social media, customer reviews, news articles, and more.
The Methodology Behind Sentiment Analysis
Several methodologies drive sentiment analysis, each leveraging distinct techniques:
Lexicon-based Approaches: Utilizing sentiment lexicons or dictionaries to assign polarities to words and phrases, enabling sentiment inference based on the frequency and context of these terms.
Machine Learning Models: Employing algorithms like Support Vector Machines, Naive Bayes, and deep learning architectures such as Recurrent Neural Networks (RNNs) or Transformer models to learn patterns and nuances in textual data for sentiment classification.
Hybrid Approaches: Merging lexicon-based methods with machine learning techniques to enhance accuracy by amalgamating rule-based sentiment analysis with the learning capabilities of ML models.
Applications of Sentiment Analysis Across Industries
Sentiment analysis boasts a diverse range of applications:
Brand and Product Monitoring: Assessing consumer sentiment towards products, services, or brands via social media, reviews, and customer feedback to shape marketing strategies and improve offerings.
Market Intelligence: Gauging public perception and trends, aiding market research and predicting consumer behavior based on sentiment shifts.
Customer Experience Enhancement: Analyzing customer feedback to enhance products or services, identify pain points, and provide personalized experiences.
Risk Management: Assessing sentiments in financial news or market reports to anticipate market movements and mitigate financial risks.
Challenges and Evolving Frontiers
Despite its remarkable utility, sentiment analysis faces challenges in comprehending sarcasm, context-dependent sentiments, and cultural nuances. The ever-evolving nature of language presents a continuous challenge in keeping sentiment analysis models updated and relevant. Yet, advancements in deep learning, particularly in contextual embeddings and transformer-based architectures, showcase promising strides in capturing nuanced sentiments and context, improving the accuracy and depth of sentiment analysis.
The Future of Sentiment Analysis
As businesses continue to harness the power of big data and NLP technologies, the importance of sentiment analysis grows exponentially. The convergence of sentiment analysis with other NLP tasks, such as aspect-based sentiment analysis and emotion detection, paves the way for more granular and insightful analyses. In conclusion, sentiment analysis stands as a transformative force in understanding human sentiments at scale, revolutionizing decision-making processes across industries. Its ongoing evolution, coupled with advancements in AI, heralds a future where deciphering emotions embedded in textual data becomes more nuanced and insightful than ever before. Understanding sentiments, after all, is not just about words—it's about decoding the human experience embedded within them. Sentiment analysis has evolved with advancements in machine learning and deep learning, enabling more accurate detection of sentiments, aspect-based sentiment analysis (identifying sentiment towards specific aspects or features), and handling nuanced sentiments in complex texts. Its applications across industries continue to expand as businesses recognize its value in understanding and leveraging customer sentiments.
Comentarios